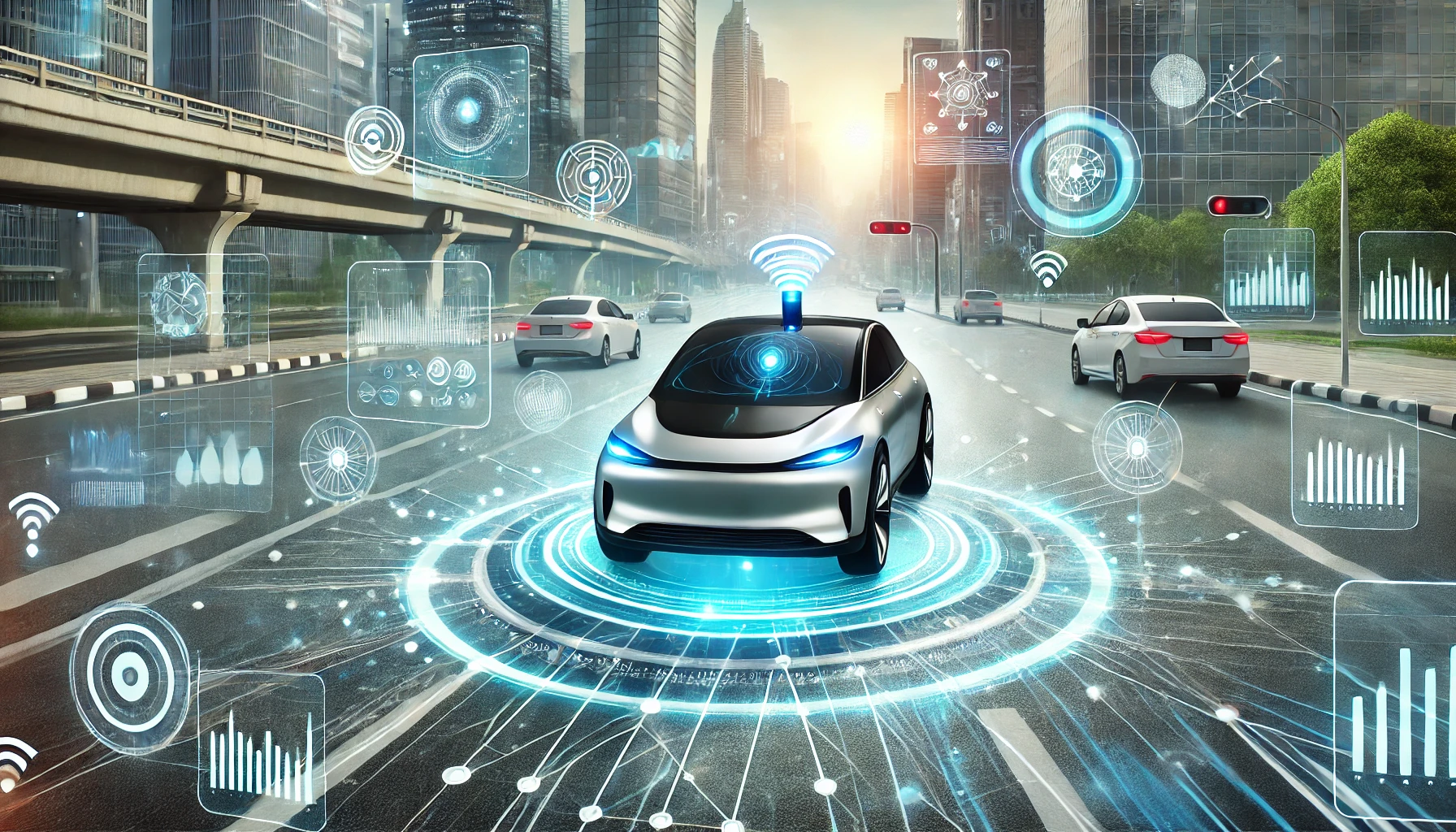
The future of transportation is no longer a distant dream. Autonomous vehicles (AVs) are transforming how we think about mobility, and at the heart of this revolution lies Artificial Intelligence (AI). But how exactly does AI drive the future of these self-driving wonders? Let’s buckle up and explore this fascinating journey.
Understanding Autonomous Vehicles
What Are Autonomous Vehicles?
Autonomous vehicles, often referred to as self-driving cars, are capable of navigating and operating without human intervention. They rely on a blend of sensors, cameras, radar, and—most importantly—AI to make real-time driving decisions.
Levels of Autonomy (SAE Levels 0-5)
The Society of Automotive Engineers (SAE) defines six levels of driving automation:
- Level 0: No automation; human controls everything.
- Level 1: Driver Assistance (e.g., adaptive cruise control).
- Level 2: Partial Automation (lane-keeping and speed control).
- Level 3: Conditional Automation (car can handle tasks, but human must intervene if needed).
- Level 4: High Automation (fully autonomous in specific conditions).
- Level 5: Full Automation (no human intervention needed, anywhere, anytime).
The Role of AI in Autonomous Vehicles
AI as the Brain Behind the Wheel
AI acts as the “brain” of autonomous vehicles, processing data from sensors, making split-second decisions, and learning from every experience. It’s the force that turns raw data into smart driving actions.
Machine Learning and Deep Learning Applications
- Machine Learning (ML): Helps cars learn from data to improve performance over time.
- Deep Learning: Mimics the human brain using neural networks to recognize patterns, objects, and complex driving scenarios.
Key AI Technologies Powering Autonomous Cars
Computer Vision
Computer vision enables AVs to “see” the environment by analyzing visual data from cameras. It helps detect traffic signs, pedestrians, lane markings, and obstacles.
Natural Language Processing (NLP)
NLP allows vehicles to understand voice commands, making interactions with passengers more natural and intuitive.
Sensor Fusion
Combining data from multiple sensors (LiDAR, radar, cameras) ensures a comprehensive understanding of the surroundings, enhancing accuracy and safety.
Path Planning Algorithms
AI-driven path planning helps AVs determine the most efficient, safe, and legal routes in real-time, adapting to dynamic road conditions.
Data: The Fuel for AI Systems
Importance of Big Data
AI thrives on data. Every second, AVs generate vast amounts of data that help improve algorithms and make smarter driving decisions.
Real-Time Data Processing
Real-time data processing enables AVs to react instantly to changing environments, ensuring swift and safe maneuvers.
AI in Decision-Making and Navigation
Object Detection and Recognition
AI identifies objects such as vehicles, pedestrians, and road signs, classifying them to make informed driving decisions.
Predictive Analytics for Safer Navigation
Predictive analytics allows AVs to anticipate potential hazards, like predicting a pedestrian’s path or an approaching vehicle’s speed.
Safety and Reliability: How AI Enhances Both
Reducing Human Error
AI eliminates common human errors like distraction, fatigue, and impaired driving, significantly reducing accident rates.
Fail-Safe Mechanisms
AI systems are designed with redundancies and fail-safe protocols to handle emergencies and system failures safely.
Challenges AI Faces in Autonomous Driving
Ethical Dilemmas and Decision-Making
Deciding how to react in life-threatening scenarios (the “trolley problem”) remains a moral and technical challenge for AI.
Handling Unpredictable Human Behavior
AI struggles to predict erratic human actions, such as jaywalking pedestrians or aggressive drivers.
Regulatory and Legal Barriers
Global laws and regulations are still catching up with AI-driven technology, posing hurdles to widespread adoption.
The Evolution of AI in the Automotive Industry
From Cruise Control to Full Autonomy
The journey began with simple cruise control systems and has evolved into sophisticated autonomous technology.
Milestones in AI Development
Key milestones include Tesla’s Autopilot, Waymo’s self-driving taxis, and advancements in AI chip technology.
Autonomous Vehicles and Smart Cities
Integration with Smart Infrastructure
AVs will seamlessly connect with smart traffic lights, IoT devices, and city-wide data networks for efficient transportation.
Traffic Management and Efficiency
AI can optimize traffic flow, reduce congestion, and improve overall city mobility.
Environmental Impact of AI-Driven Vehicles
Reducing Carbon Footprint
Electric AVs combined with AI-driven efficiency reduce greenhouse gas emissions.
Sustainable Transportation Solutions
AI enables ride-sharing models that decrease the number of vehicles on the road, promoting sustainability.
The Future of Mobility with AI
Autonomous Ride-Sharing Services
Companies like Uber and Lyft are exploring autonomous fleets, offering cost-effective, eco-friendly transportation.
AI in Public Transport Systems
Buses, trains, and shuttles are becoming smarter with AI, offering safer and more reliable public transport.
AI and the Passenger Experience
Personalized In-Car Environments
AI customizes climate, music, and seating preferences for a tailored passenger experience.
Voice Assistants and Smart Interfaces
Advanced voice assistants provide hands-free control, making rides more convenient and interactive.
The Competitive Landscape
Key Players in AI-Driven Autonomous Vehicles
Tech giants like Tesla, Google’s Waymo, Apple, and traditional automakers are leading the race.
Collaborations and Innovations
Partnerships between automakers, AI companies, and governments accelerate technological advancements.
Conclusion
AI is the backbone of autonomous vehicles, steering the future of mobility towards safety, efficiency, and sustainability. As technology evolves, so will our roads, cities, and lifestyles, making the dream of fully autonomous vehicles a reality.