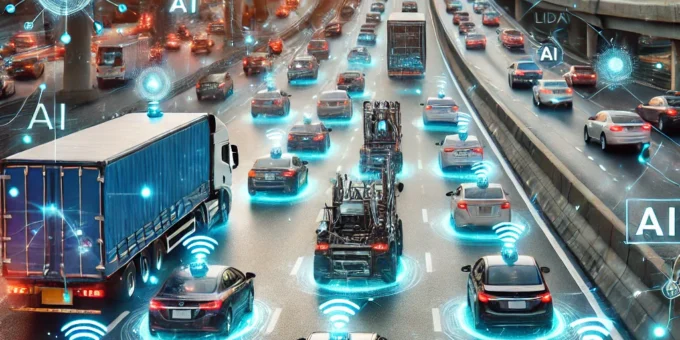
Introduction
Artificial Intelligence (AI) and machine learning are transforming the transportation industry, revolutionizing how we travel, commute, and transport goods. Autonomous vehicles (AVs) are at the forefront of this technological revolution, using AI to analyze traffic conditions, detect objects, and make real-time driving decisions.
With companies like Tesla, Waymo, and NVIDIA pioneering self-driving technology, AI-powered transportation is becoming a reality. But how exactly does AI enable vehicles to drive autonomously? What role does machine learning play in training cars to navigate the roads safely? This article explores the impact of AI in self-driving vehicles, its core technologies, and what the future holds for this groundbreaking innovation.
What Are Autonomous Vehicles?
Autonomous vehicles, also known as self-driving cars, are equipped with AI, sensors, and machine learning models that allow them to operate without human intervention. These vehicles rely on a combination of LiDAR, radar, cameras, and neural networks to perceive their environment and make driving decisions.
The concept of self-driving cars dates back decades, but it wasn’t until the 21st century that AI and computing power made full autonomy a possibility. Today, major tech firms and automakers are in a race to develop fully autonomous vehicles, with the potential to redefine urban mobility and transportation systems.
How AI Powers Autonomous Vehicles
AI is the driving force behind self-driving cars, enabling them to:
- Process sensor data to detect traffic, pedestrians, and road signs.
- Make real-time decisions about acceleration, braking, and lane changes.
- Learn from past experiences to improve driving behavior.
- Navigate routes using AI-powered mapping and GPS.
AI in autonomous vehicles is primarily built on machine learning, deep learning, and computer vision to create a seamless and efficient driving experience.
Machine Learning in Self-Driving Cars
Machine learning (ML) is a subset of AI that allows self-driving cars to learn from vast amounts of data collected from real-world driving. Through neural networks and reinforcement learning, vehicles continuously improve their ability to:
- Recognize objects (cars, pedestrians, cyclists).
- Predict movement patterns of other vehicles.
- Respond to changing road conditions (weather, construction, detours).
For example, Tesla’s Autopilot and Waymo’s self-driving software rely on millions of miles of driving data to enhance their AI algorithms. The more data the system processes, the better the car gets at driving autonomously.
Levels of Vehicle Autonomy
The Society of Automotive Engineers (SAE) defines six levels of automation:
- Level 0: No automation (human driver required).
- Level 1: Driver assistance (adaptive cruise control, lane-keeping assist).
- Level 2: Partial automation (Tesla Autopilot, GM Super Cruise).
- Level 3: Conditional automation (self-driving in specific conditions).
- Level 4: High automation (fully autonomous in designated areas).
- Level 5: Full automation (no human intervention required).
Currently, most autonomous vehicle technology is at Level 2 or Level 3, but advances in AI are pushing toward Level 5 full autonomy.
AI and Real-Time Decision Making
AI-powered self-driving cars use real-time decision-making systems to react to their surroundings instantly. These systems rely on:
- Computer vision to identify obstacles and road markings.
- Path planning algorithms to determine the safest route.
- Sensor fusion to combine data from LiDAR, radar, and cameras.
By continuously learning and adapting, AI ensures that autonomous vehicles drive more safely and efficiently than human drivers.
Safety and Security in AI-Driven Vehicles
AI improves road safety by eliminating human errors, which cause over 90% of traffic accidents. AI-powered features include:
- Collision detection and avoidance to prevent accidents.
- Predictive analytics to anticipate dangerous situations.
- Cybersecurity measures to protect against hacking threats.
While AI reduces accidents, concerns about data privacy and cybersecurity remain challenges that need to be addressed.
Autonomous Vehicles in Public Transport
AI-driven public transport systems are reshaping urban mobility with:
- Self-driving taxis (Waymo One, Cruise AV).
- Autonomous buses for smart cities.
- On-demand ride-sharing AI algorithms for efficient commuting.
These AI-powered solutions reduce congestion, emissions, and transportation costs, making urban travel more efficient.
Future Trends in AI and Autonomous Vehicles
The future of AI in transportation will include:
- Full adoption of Level 5 self-driving cars.
- AI-powered smart traffic systems for better road management.
- Autonomous delivery trucks and drones.
- Integration of AI with 5G for instant vehicle communication.
AI and autonomous vehicles are set to redefine global transportation, making roads safer and travel more efficient.
Machine learning and AI are revolutionizing autonomous vehicles, making transportation safer, smarter, and more efficient. As AI technology continues to evolve, self-driving cars will become a mainstream reality, transforming how we move.