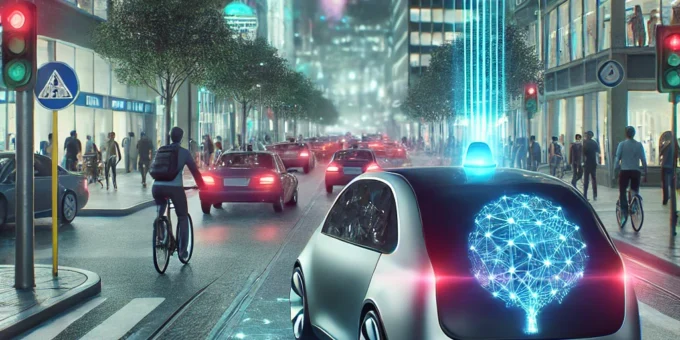
Introduction
The rise of AI in autonomous vehicles is revolutionizing the automotive industry, making self-driving cars a reality. This technology is a game-changer, blending machine learning, sensor technology, and real-time decision-making to create safer and more efficient transportation systems. Over the past decade, AI has advanced rapidly, enabling vehicles to interpret their surroundings, make intelligent decisions, and navigate complex road conditions without human intervention.
Autonomous driving has the potential to reduce accidents, improve traffic flow, and transform urban mobility. But how exactly does AI power self-driving cars? What challenges still need to be addressed before full autonomy becomes mainstream? In this article, we’ll break down the core technologies behind self-driving vehicles, explore the role of AI, and discuss what the future holds for this groundbreaking innovation.
What is AI in Autonomous Vehicles?
AI in self-driving cars refers to the use of machine learning, deep learning, and neural networks to enable vehicles to operate without human drivers. The AI system processes sensor data, recognizes objects, predicts behavior, and makes split-second driving decisions.
Key Components of AI in Self-Driving Cars
- Perception Systems – AI analyzes data from cameras, LiDAR, radar, and ultrasonic sensors to detect obstacles, road signs, and pedestrians.
- Decision-Making Algorithms – Advanced AI algorithms decide when to accelerate, brake, or change lanes based on traffic conditions.
- Path Planning and Navigation – AI integrates real-time GPS, HD maps, and traffic updates to optimize routes.
- Machine Learning Models – AI continuously learns from new data, improving its driving skills over time.
Levels of Vehicle Autonomy
The Society of Automotive Engineers (SAE) has defined six levels of vehicle automation:
- Level 0: No automation, human driver in full control.
- Level 1: Driver assistance (adaptive cruise control, lane-keeping assist).
- Level 2: Partial automation (Tesla’s Autopilot, GM’s Super Cruise).
- Level 3: Conditional automation (AI can drive in certain conditions but needs human override).
- Level 4: High automation (fully self-driving but within geofenced areas).
- Level 5: Full autonomy (AI can drive anywhere without human intervention).
Currently, most self-driving systems are at Level 2 or 3, but industry leaders are working toward Level 5 autonomy.
How AI Powers Self-Driving Cars
Self-driving cars rely on a combination of machine learning, deep learning, and neural networks to interpret the world around them.
Machine Learning in Autonomous Vehicles
Machine learning allows vehicles to:
- Detect objects such as pedestrians, cyclists, and road signs.
- Predict movement patterns of other vehicles.
- Adapt to real-world driving scenarios by continuously learning from new data.
Deep Learning and Neural Networks
Deep learning uses AI models that mimic the human brain, processing vast amounts of driving data to improve accuracy in:
- Traffic sign recognition
- Lane detection
- Collision avoidance
Sensor Technology in Autonomous Vehicles
AI-driven cars require advanced sensors to perceive their environment accurately. The primary types of sensors include:
- LiDAR (Light Detection and Ranging) – Provides 3D mapping of surroundings.
- Radar – Detects objects in poor weather conditions.
- Cameras – Recognize traffic signals, lane markings, and pedestrians.
- Ultrasonic Sensors – Assist in parking and detecting nearby obstacles.
These sensors work together to build a 360-degree awareness of the car’s surroundings.
Decision-Making Systems
AI in self-driving cars uses reinforcement learning and probabilistic algorithms to make real-time driving decisions.
- Path Planning Algorithms: Determine the most efficient driving route.
- Risk Assessment AI: Evaluates potential hazards and takes corrective actions.
- Traffic Prediction Models: Predict congestion and suggest alternative routes.
By integrating AI with cloud computing, self-driving cars can also share and learn from real-time traffic data.
Future Trends in AI and Autonomous Vehicles
The future of AI-powered self-driving cars includes:
- Widespread adoption of Level 5 autonomy
- AI-integrated smart traffic systems
- Enhanced V2X (Vehicle-to-Everything) communication
- AI-driven fuel efficiency improvements in electric vehicles
With ongoing advancements, self-driving cars will become a mainstream mode of transportation, transforming urban mobility worldwide.
Final Thoughts
AI in autonomous vehicles is at the forefront of transportation innovation, reshaping how people and goods move. While challenges such as regulations, cybersecurity, and AI ethics remain, the future of self-driving cars looks promising. With continuous advancements in machine learning, sensor technology, and AI decision-making, the dream of fully autonomous transportation is closer than ever.
Inbound and Outbound Link Suggestions
- Internal Link: “Read more about AI and Machine Learning in Transportation”
- External Link: “Explore SAE’s official standards on vehicle automation (sae.org)”